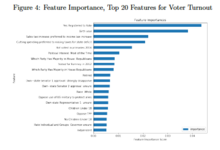
Abstract
Machine learning is becoming increasingly prevalent in political science research. Improving the accuracy of outcomes, refining measurements of complex processes, addressing non-linearities in data and introducing new kinds of data may be achieved using machine learning. Despite the possible uses of machine learning, a clear understanding of how to use these tools and their pitfalls is still needed. This article provides a foundational guide to machine learning and illustrates how these methods can advance political science research. We address the pitfalls of these methods as well as the specific concerns for using machine learning with social data. Finally, we demonstrate how machine learning can help understand voter turnout through an application of methods with survey data on the 2016 election.